How to Evaluate AI-Powered Products: A Decision-Maker’s Guide to Success?
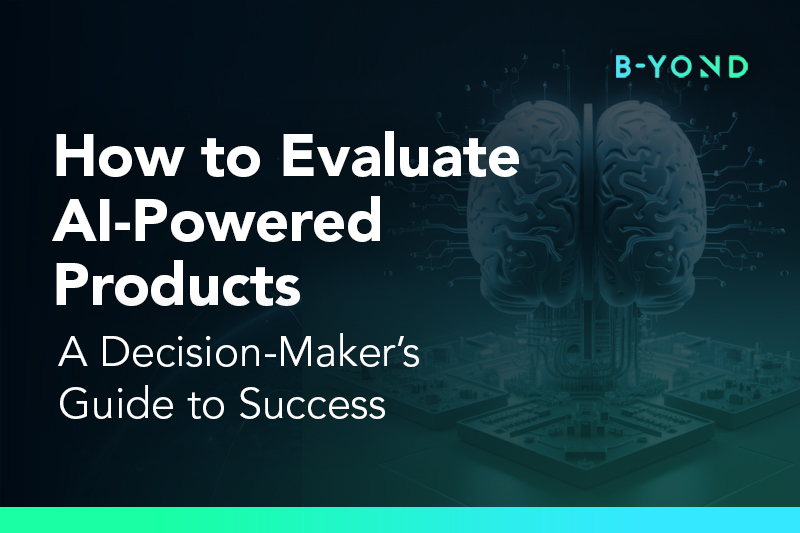
How to Evaluate AI-Powered Products: A Decision-Maker’s Guide to Success?
Introduction:
This blog is designed for telecommunications decision-makers tasked with ensuring customer experience, network reliability, and operational efficiency. If you're exploring AI-powered products for network operations, this blog is for you.
Imagine this: A major telecommunications provider tested an AI product on their most complex network issues. When the AI initially failed to identify the root causes, the product was dismissed. But during a major outage, the team spent several hours manually diagnosing the problem. Out of curiosity, they reran the same scenario through the AI product afterward—within minutes, it provided the precise diagnosis. This raises the question: are we too focused on AI perfection, causing us to overlook its real-world value?
We understand the challenges you face. Evaluating new AI products requires time and effort, especially when it involves fast-evolving technologies that may not be well understood. There’s often natural resistance to changing established workflows, particularly in highly constrained, security-driven environments. On top of that, selecting the wrong product carries significant long-term risks. This blog will help you navigate these challenges, providing insights into how to fairly and effectively evaluate AI products, reducing friction and minimizing risks associated with adoption.
1. AI Unrealistic Expectations
Many decision-makers come to AI solutions expecting them to be silver bullets, offering flawless performance in every situation. This often leads to disappointment when AI doesn't immediately deliver perfect results. AI is not about being 100% right all the time, but rather about consistently improving and enhancing decision-making capabilities. Understanding its limitations is key: AI can reduce time to resolution, handle vast amounts of data, and detect patterns far beyond human capability—but it’s not immune to occasional errors or challenges in complex edge cases. Setting realistic expectations about AI's evolving nature is critical to avoid misalignment between what the technology offers and what leadership anticipates.
2. AI in Real-Life Scenarios
In real-world telecom environments, AI is often tested under a range of unpredictable conditions—live networks with heavy traffic, unexpected failures, and a myriad of potential configurations. AI’s true value lies in how it performs in these dynamic environments, where the stakes are high, and decisions need to be made quickly. Decision-makers need to assess how AI adapts to day-to-day network operations, handles large-scale data, and integrates with existing workflows. The key is to evaluate how AI can enhance productivity in real-world applications, whether that’s identifying root causes faster, automating routine diagnostics, assisting your engineers or providing predictive maintenance insights.
3. AI in Unseen Situations
One of the biggest challenges in evaluating AI is understanding how it performs in situations it has never encountered before. Networks are constantly evolving, and new types of failures or configurations can emerge. A robust AI product should be able to generalize beyond its training data, offering meaningful insights even when it faces something new. Decision-makers must assess AI's resilience in such scenarios—how well it can identify novel patterns, flag anomalies, and offer plausible root causes when dealing with unknowns. Testing AI under unseen circumstances helps reveal its adaptability and ensures it can handle future challenges.
4. AI-Chosen Guide to Succeed
Success with AI-powered products hinges on a well-thought-out approach to evaluation and deployment. Decision-makers should focus on selecting AI products that align with their specific business goals and operational needs. This involves creating a clear set of success criteria: Is the goal faster diagnostics? Improved customer experience? Greater network uptime? AI solutions that are chosen based on these defined objectives, and that have a measurable impact on key metrics, will yield the most value. They should build a flexible evaluation playground that enable them to safely experiment, increment faster, integrate seamlessly with existing processes while always assuming that AI might fail. Decision-makers should involve 0-to-1 teams to learn, execute, gather early feedback and ensure AI products are tested using simulated, real, unexpected, live data to gauge accuracy, performance, integration and risks.
Conclusion:
Embracing AI in telecommunications isn't just about adopting new technology; it's about transforming how your organization operates and competes. By managing expectations, evaluating AI in real-world and unforeseen scenarios, and strategically selecting solutions aligned with your goals, you can harness AI's full potential.
Remember the provider in our opening story—their initial dismissal of AI overlooked significant potential. By approaching AI with an open mind and accepting shortfalls, you can avoid similar pitfalls, drive meaningful improvements alongside human expertise in your network operational efficiency, and customer experiences.