Join the Largest Network Operators Scaling Network Protocol Analysis with B-YOND
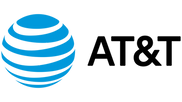
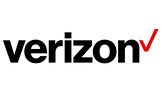
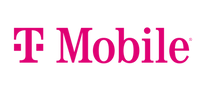
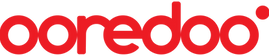
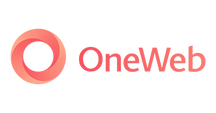
Analyzed
Identified
B-Yond brings machine learning and artificial intelligence to telecom network operators all over the world.
More reliable and faster 5G rollouts
Automated packet analysis
Intelligent root cause identification
Network failures knowledge bases
Seamless troubleshooting of PCAPs
Automatic resolution of network elements' role, name, and topology.
Instant call flow classification as success, warning and failure.
Identify errors and isolate the network
function where it occurred.
Illustrate the sequence of messages on a ladder diagram, with protocol and network element level errors, and golden callflow comparison.
AGILITY has transformed the network testing landscape for a Tier 1 North American Operator by streamlining processes and significantly reducing time-to-market for new features.By utilizing Continuous Validation (CV) and Continuous Testing (CT) with a comprehensive AI Repository, B-Yond has achieved remarkable results in network quality and efficiency, enabling the operator to stay ahead in the competitive market.
An average test cost reduction of 50%.
Time-to-Market for new features accelerated.
Test life cycles decreased from hours to minutes.
10x increase in test capacity improves network quality & hastens resolution times.
Leverage AI and ML to reduce the time and costs of repeated investigations on recurring patterns of network failures.